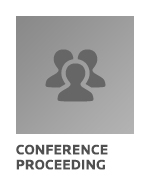
C058 — Automatic Segmentation of Building Envelope Point Cloud Data Using Machine Learning
Click here to purchase
About 50% of buildings in the US were constructed before energy codes were introduced. Modular overclad panel retrofits, in which a new envelope is constructed over the existing building, are a promising solution given that it minimizes occupant disruption and shortens construction time at the jobsite. Current state-of-the-art retrofit panel layout and dimensioning consists of three steps: 1) 3D point cloud data generation of the building envelope using commonly available surveying equipment, 2) manual segmentation of 3D point cloud data by a trained professional to identify and dimension window openings, door openings, and other architectural features, and 3) modular panel layout optimization and dimensioning by an architect or engineer. Among these steps, the second one remains the most difficult and costly because it is very labor-intensive. We propose a methodology to automatically label 3D point cloud data to reduce the time and expense spent in manual segmentation. Machine learning methods were employed to classify the point cloud data into distinct groups, each of which corresponds to different features of the building envelope. After classification, a segmentation algorithm was developed to perform boundary detection and separate the components of the façade. Finally, the algorithm returns the relative positions and dimensions of the features in the building envelope. The measurements obtained with the proposed automated method were compared against the actual dimensions to determine the overall algorithm accuracy. The proposed algorithm can then be used to reduce manual efforts for 3D point cloud labeling before modular panel layout optimization is performed.
Product Details
- Published:
- 2022
- Number of Pages:
- 8
- Units of Measure:
- Dual
- Product Code(s):
- DBldgsXV-C058