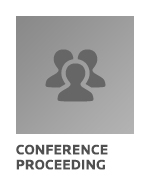
C076 — Evaluation of the Applicability of ASHRAE’s Damage Function Model to Predict Moisture Severity of the Climate for Canadian Locations
Click here to purchase
Hygrothermal simulation tools are commonly used to assess the moisture performance of building envelope components. Owing to the computational costs required to complete simulations over the long-term, one approach to reduce simulation time when undertaking hygrothermal design analysis, is to select representative year(s) amongst sets of long-term climate data. To properly select these moisture reference year(s), a method is required to rank the climate years in terms of their moisture severity. To this end, several methods have been proposed in the literature, amongst which is the damage function method as reported in ASHRAE Project Report RP-1325. In this method, a stepwise regression model was developed to predict the damage function, as characterized by the RHT-index (integral of (Temperature – 0) (Relative Humidity – 70%)), in an OSB layer of a wood-framed wall as a function of several average yearly climate parameters for a North facing wall. The model was calibrated using climate and simulation data for eight cities in USA and validated for four cities comprising Winnipeg, Canada. The method was found to be the most consistent and accurate amongst all ranking methods as have been evaluated. The objective of this paper was to: (1) evaluate the ASHRAE method for several Canadian locations; (2) determine whether the original model can be recalibrated in the event it is shown to be deficient; and (3) explore the potential of improving the model using other approaches such as the Partial Least Squares Regression (PLSR). The results suggest that for some Canadian locations, as were investigated in this study, the use of the original model may not be appropriate for prediction. However, the model was shown to perform better after it was recalibrated for Canadian locations but without improvement in ranking performance. Furthermore, the damage function model can be improved by using PLSR in terms of prediction and ranking.
Product Details
- Published:
- 2022
- Number of Pages:
- 9
- Units of Measure:
- Dual
- Product Code(s):
- DBldgsXV-C076